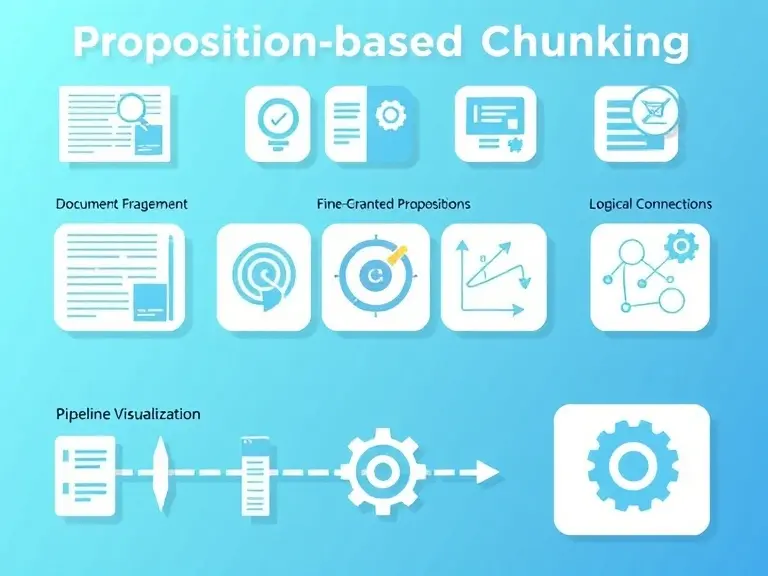
Introduction
In the rapidly evolving world of Natural Language Processing (NLP), Retrieval-Augmented Generation (RAG) has emerged as one of the most powerful techniques for improving the accuracy and context-awareness of large language models (LLMs). By combining retrieval mechanisms with generative capabilities, RAG systems should be able to fetch relevant chunks of information and leverage them to generate precise, contextually enriched responses.
However, one of the most challenging aspects of building efficient RAG systems is chunking — the process of splitting large documents into smaller, semantically coherent sections to improve retrieval quality. While traditional chunking methods such as splitting by paragraphs or fixed-length blocks of text can work, they often miss out on capturing fine-grained semantic structures. Enter Proposition-Based Chunking, an advanced strategy designed to optimize chunking by breaking text into logical propositions, leading to higher-quality retrieval and generation in RAG systems.
In this blog post, we'll dive into the details of proposition-based chunking, explore its implementation in the LangChain framework, and discuss how it can significantly improve retrieval in RAG-based applications.
Why chunking matters in RAG
Before we explore proposition-based chunking, let's first understand the importance of chunking in a RAG pipeline:
Efficient Retrieval: Large documents are often too unwieldy to retrieve all at once. By breaking them into smaller, semantically meaningful chunks, we can focus retrieval on the most relevant pieces of information.
Context Preservation: Overly large or random chunks can obscure the contextual relationships between ideas. Well-crafted chunks ensure that responses generated by the model are both relevant and coherent.
Performance Optimization: Smaller, logically segmented chunks allow for faster search and retrieval operations, reducing computational costs and improving response time.
Traditional methods like paragraph-based or fixed-length chunking don't always provide optimal results because they often miss the subtle logical boundaries between ideas. This is where proposition-based chunking comes into play.
Proposition-Based Chunking?
Proposition-based chunking is a more granular chunking strategy that divides a document into its core propositions — distinct units of meaning or assertions. These propositions are often more fine-grained than sentences and offer a more natural division for semantic retrieval. By capturing the underlying logic and assertions made in the text, proposition-based chunking allows retrieval mechanisms to return highly relevant chunks.
In essence, instead of breaking text into arbitrary sections, proposition-based chunking focuses on the smallest meaningful components of text — propositions that reflect specific ideas, claims, or facts. This leads to higher retrieval precision in RAG systems since each chunk directly corresponds to a logically complete thought.
How Proposition-Based Chunking Works in LangChain
To implement proposition-based chunking within a RAG system, you can leverage the LangChain framework, which provides tools for building pipelines involving language models, retrieval components, and custom chunkers. Below is an overview of how we can implement proposition-based chunking using LangChain and integrate it with a retrieval-augmented generation pipeline.
Step 1: Text Preprocessing and Proposition Extraction
First, the text is preprocessed by extracting logical propositions. Using a combination of natural language understanding models and extraction chains (provided by LangChain), we can break the text into meaningful propositions. Here's how you might implement it:
from langchain.chains import create_extraction_chain_pydantic
from langchain_openai import ChatOpenAI
from langchain_core.pydantic_v1 import BaseModel
class Sentences(BaseModel):
sentences: List[str]
# Instantiate the LLM and extraction chain
llm = ChatOpenAI(model='gpt-3.5-turbo')
extraction_chain = create_extraction_chain_pydantic(pydantic_schema=Sentences, llm=llm)
def get_propositions(text):
# Using LangChain's extraction chain to extract propositions from the text
return extraction_chain.invoke(text)["text"][0].sentences
Step 2: Grouping Propositions into Semantically Coherent Chunks
Once the propositions have been extracted, we can group them into semantically coherent chunks. Here, we use an Agentic Chunker that intelligently assigns propositions to relevant chunks based on their semantic meaning:
from agentic_chunker import AgenticChunker
# Initialize the Agentic Chunker
ac = AgenticChunker()
propositions = get_propositions(text)
# Add propositions to the chunker
ac.add_propositions(propositions)
# Retrieve and print the final chunks
chunks = ac.get_chunks(get_type='list_of_strings')
print(chunks)
The Agentic Chunker helps ensure that each chunk is meaningful and contextually consistent, based on the semantic similarity of propositions. When a new proposition is added to an existing chunk, the chunk’s title and summary are automatically updated using the language model, ensuring that the metadata remains relevant.
Step 3: Creating a RAG System with Proposition-Based Chunking
Finally, once the propositions have been chunked, we integrate them into the RAG pipeline. We use the Chroma vector store from LangChain to index these chunks and enable fast, vector-based retrieval.
from langchain.docstore.document import Document
from langchain_community.vectorstores import Chroma
from langchain_community import embeddings
# Create document objects from the chunks
documents = [Document(page_content=chunk, metadata={"source": "local"}) for chunk in chunks]
# Embed the documents and store them in the vector store
vectorstore = Chroma.from_documents(
documents=documents,
collection_name="agentic-chunks",
embedding=embeddings.ollama.OllamaEmbeddings(model='nomic-embed-text'),
)
# Perform retrieval and generate answers
retriever = vectorstore.as_retriever()
result = retriever.retrieve("What is the use of text splitting?")
print(result)
This setup completes a fully functional RAG pipeline that uses proposition-based chunking to enhance the quality of information retrieval.
Benefits
Increased Precision: Since each chunk is built around a core proposition, retrieval is much more likely to return the most relevant information, leading to more precise answers.
Improved Contextuality: By focusing on semantically related propositions, chunks remain contextually coherent, which ensures that the generative model produces meaningful and accurate outputs.
Better Scalability: Proposition-based chunking divides documents into smaller, more manageable pieces, improving the efficiency of vector searches and retrieval in large corporation.
Dynamic Chunking: The ability to dynamically update chunk summaries and titles ensures that metadata remains relevant, even as new propositions are added.
Conclusion
Proposition-based chunking is an advanced chunking strategy that offers significant improvements over traditional methods, particularly in retrieval-augmented generation systems. By focusing on core propositions, this approach enhances both the precision and context of the retrieved information, enabling RAG systems to provide more accurate, contextually aware, and informative responses. When integrated with tools like LangChain, proposition-based chunking becomes an invaluable asset for NLP applications requiring highly granular and semantically meaningful retrieval.
As the field of NLP continues to evolve, techniques like proposition-based chunking will likely become standard practice for building intelligent, scalable, and high-performing information retrieval systems.