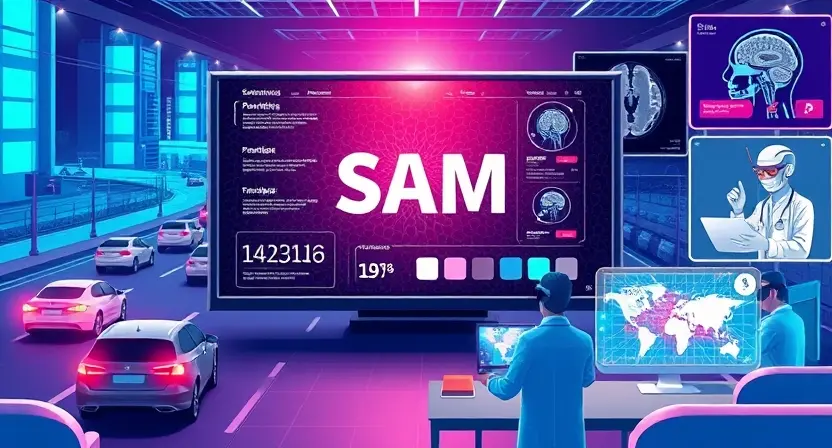
The world of Artificial Intelligence (AI) and computer vision has seen rapid advances, making tasks that were once time-consuming and complex, now more accessible and automated. A powerful new player in this space is SAM, the Segment Anything Model, which has the potential to revolutionize how we approach object detection, image segmentation, and analysis. SAM aims to break new ground in enabling machines to understand and interact with the visual world in ways previously thought impossible.
SAM (Segment Anything Model)
SAM (Segment Anything Model) is a cutting-edge model developed to solve one of the most complex problems in computer vision—image segmentation. Image segmentation is the process of partitioning an image into different segments or regions to identify and differentiate objects within the image. It’s one of the foundational building blocks of AI-driven applications like autonomous vehicles, medical imaging, robotics, and augmented reality.
While traditional segmentation models are highly specialized, requiring training on specific datasets for each task (e.g., segmenting cars in street scenes or detecting tumors in medical scans), SAM is designed to generalize across tasks. Its main goal is to segment any object, from any image, in any context—hence its name, "Segment Anything."
Key Features of SAM
SAM introduces several key innovations that set it apart from existing models:
Generalizability: SAM doesn’t need to be retrained for specific use cases. Instead, it can take any image and accurately segment objects, even those it hasn’t seen before.
Prompt-based Segmentation: One of SAM’s most impressive capabilities is its ability to respond to different types of prompts. For example, users can provide:
- Points: By pointing to a pixel in an image, SAM can identify and segment the object.
- Boxes: A bounding box drawn around an object can tell SAM to segment everything within the box.
- Text Prompts: SAM can also be prompted using natural language, where the user can specify objects in text form (e.g., “segment the cat in the image”).
Real-time Performance: Unlike traditional models that often take time to process, SAM is optimized for speed, delivering segmentation results in near real-time.
Large-scale Training: SAM is pre-trained on an enormous dataset that contains diverse images and objects, allowing it to accurately detect and segment even obscure or complex objects. This makes SAM adaptable across various domains without needing retraining on task-specific data.
How SAM Works: The Architecture
SAM is built upon a transformer-based architecture, one of the most successful paradigms in modern AI. Here's a high-level overview of how SAM processes an image:
Image Encoding:
SAM takes an input image and uses a powerful image encoder, typically based on convolutional neural networks (CNNs) or vision transformers, to extract deep feature representations of the image.
Prompt Encoding:
Depending on the type of prompt (point, box, or text), SAM processes the user input and creates an embedding representing that prompt.
Mask Generation:
SAM fuses the image and prompt embeddings and feeds them into a transformer to predict a segmentation mask. This mask identifies which regions of the image correspond to the object of interest based on the provided prompt.
Object Classification:
While SAM is primarily focused on segmentation, it can also be combined with other models (such as those trained on the ImageNet dataset) to classify objects in the segmented regions.
SAM’s Impact on Various Industries
The versatility and power of SAM open up new possibilities in a wide range of industries. Here’s a closer look at how SAM could transform some key sectors:
Autonomous Vehicles:
Autonomous driving relies on accurate and real-time object detection to make safe decisions. SAM’s ability to segment objects—like pedestrians, other vehicles, and road signs—in various lighting and weather conditions could significantly improve the safety and reliability of self-driving cars. With SAM, vehicles can detect and classify objects faster, even in highly complex environments like busy intersections or highways.
Medical Imaging:
In the healthcare industry, SAM could make significant contributions to medical imaging by enabling precise segmentation of organs, tissues, or tumors in scans such as MRIs or X-rays. With SAM’s generalizability, it could handle various medical tasks, from identifying abnormalities in brain scans to segmenting tumors for treatment planning.
Augmented Reality (AR) and Virtual Reality (VR):
SAM can enhance AR/VR experiences by accurately segmenting objects in real time, allowing virtual objects to interact seamlessly with the real world. For example, SAM could be used in AR shopping apps where a user can “try” furniture in their room by segmenting real-world objects like walls and floors.
Robotics:
Robotic systems often struggle with recognizing and interacting with their environment. SAM enables robots to quickly identify and understand different objects in their surroundings, which is crucial for tasks like picking and placing items, navigating through complex environments, or even assisting in surgeries.
Environmental Monitoring:
Satellite imagery and drone footage are increasingly used to monitor environmental changes, detect deforestation, or track wildlife. SAM can help researchers easily segment and classify different land-use types, water bodies, or vegetation cover in remote sensing images, providing more accurate and detailed analyses.
Why is SAM a Game-Changer in AI?
SAM stands out because of its ability to work out of the box on various segmentation tasks without needing extensive retraining. This flexibility allows it to be deployed in settings where time, budget, or domain-specific data are limited. Additionally, its prompt-based segmentation introduces a new level of interactivity, allowing both experts and non-experts to guide and refine the model’s results.
Another compelling aspect of SAM is its potential for unsupervised and self-supervised learning. By leveraging massive amounts of unlabeled data, SAM learns how to identify objects without relying on human-labeled datasets, which is crucial for scaling AI applications to new domains and industries.
Challenges and Limitations
While SAM offers impressive capabilities, it’s not without challenges. Some areas where further improvements could be made include:
Fine-grained segmentation:
SAM may struggle with objects that have fine details, such as hair strands or transparent materials.
Ambiguous prompts:
While SAM can handle multiple types of prompts, some complex scenarios (e.g., overlapping objects or highly occluded objects) may still pose challenges.
Computational Resources:
Running SAM in real-time for high-resolution images may require substantial computational power, which could limit its deployment in resource-constrained environments.
Conclusion
SAM represents a major leap forward in AI and computer vision. Its ability to generalize across domains, combined with its intuitive prompt-based interface, makes it a versatile tool that can be applied across industries ranging from healthcare and autonomous vehicles to robotics and AR/VR. As SAM and similar models continue to evolve, we can expect to see even more sophisticated AI-powered systems capable of understanding and interacting with the visual world like never before.
The future of SAM lies in its integration with other AI models and technologies, further pushing the boundaries of what AI can achieve in vision-related tasks. With its ability to segment anything, SAM is set to unlock new possibilities and use cases that could redefine how we interact with AI in our everyday lives.
In the coming years, SAM and other generalized models may become standard components of AI systems, bringing the power of computer vision to more industries, research fields, and real-world applications than ever before.